Roughly $280 billion of new funding was authorized to boost research and production of semiconductors in the US under the CHIPS and Science Act in 2022 - an amount greater than the inflation-adjusted initial spending to create the US Interstate Highway System. The legislation was just one of multiple acts engineered to subsidise and support emerging technologies in the US that are bound to have seismic impacts on the labor market. It signifies how swift changes in new and emerging technologies have the potential to profoundly change the demand for skills and the structure of work. Here AI has the potential to be more disruptive than any other technological development since the industrial revolution.
The US is not alone. Countries across the globe are trying to understand the potential for AI to affect their workforce and economic activity. IPSOS, Group SA, a multinational market research company with headquarters in France, recently attempted to gauge people’s feelings towards AI across the world through a survey across 31 countries and interviews with a small cohort of AI leaders (Global Views on AI 2023). However although extensive, the data retrieved shares the limitations common to all surveys. The OECD’s most recent Employment Outlook devotes six out of seven chapters to understanding the impact of AI on the workforce. But the OECD also notes that “No comprehensive method exists by which to track and compare AI R&D funding across countries and agencies.” 1 Not surprisingly, the inability to track, let alone compare AI R&D funding, means that it is difficult to make predictions about the R&D induced global labor market consequences.
The lack of a comprehensive method, and the resultant uncertainty about impact, is a clarion call to action. There are many challenges that need to be addressed. A partial list would include the following: a) a lack of a common definition of AI; b) a lack of information about the needed AI capabilities and how they will change; c) mapping AI capabilities to occupational skillls; and d) an inability to measure the impact of AI on job replacement or job augmentation.
Fortunately, there is hope, with new partnerships being established in the US by universities, federal, and state agencies. A new data infrastructure is being developed at the Institute for Research on Innovation and Science (IRIS) at the University of Michigan, joint with Ohio State University, in the United States, funded by the federal US National Science Foundation (NSF). The pilot joins up existing data using university and state sources to trace how scientific innovation translates to the labor market 2. The NSF, which was been charged with the regional implementation of the CHIPS and Science investments, is funding the pilot precisely because it needs “innovative tools to accurately assess the impact of these investments across the U.S. 3
How bad is the problem?
The lack of data results in conflicting information. Some reports have warned of apocalyptic takeovers of the job market for many professions. Indeed, a heavily cited report by Goldman Sachs 4 predicted that AI could replace 300 million jobs. But the same BBC report that cited the Goldman Sachs prediction quoted the future-of-work director at Oxford University, Carl Benedikt Frey as saying “The only thing I am sure of is that there is no way of knowing how many jobs will be replaced by generative AI”. Simply put, as the former US Federal CIO, Suzette Kent, said “we lack useful information for informing strategic decisions for national workforce matters.”
So just how much of a problem is it that there is no information on how investments in science and technology affect the labor market? Why should we worry if we cannot accurately predict the impact of AI on workers, firms, and jobs? One reason is to avoid the mistakes of the past, in which both workers and firms have borne the consequences of bad information. Just in recent history, digitization and globalization resulted in a devastating loss of jobs in many countries. And geographic inequality soared as jobs in the midwestern and northeastern urban centers were lost and a service economy on the coasts burgeoned. Efforts to reduce the loss of jobs and earnings came too little, too late 5 6. Another reason is to make evidence based policy recommendations. For example, the US National AI Research Resources Taskforce, which was directly charged by the President and Congress with recommending ways to invest in AI research to strengthen and democratize the U.S. AI innovation ecosystem did not have joined up data between science investment and the workforce to inform their final recommendations. 7
In other words, governments need more timely, local, and actionable data so that they can understand changes in the tasks that employers need performed, which types of jobs and firms will be affected, and where. Concomitantly, data will be needed about the effects of AI on different population groups and different geographic areas so that the costs of change are not unfairly distributed. Armed with such information, policy makers can make investments that mitigate or counteract negative impacts and workers can be trained in the new necessary skills and matched with the firms that need them. But the swift pace of change in AI means that the urgency to create timely, local, and actionable labor market information to guide these investments has never been greater.
A new approach
The IRIS approach, called the “Industry of Ideas” builds on the “economics of ideas” framework for which Paul Romer received the 2018 Nobel Prize in Economics”. 8 9. People who create ideas – new technologies – that can be reused, form the foundations of new industries. In other words, “the discovery of new ideas lie at center of economic growth…” (Charles Jones describing Paul Romer’s conceptual framework) 10.
The project recognizes that, as Robert Oppenheimer said “the best way to transmit knowledge is to wrap it up in a human being”. 11 It uses people-centric methods for following the movement of ideas from investments in research into the marketplace. The approach identifies businesses that employ people with deep skills in AI and other emerging technology areas and developing early, never-before-available indicators that can provide alerts associated with potential impacts on current and future workforce. Initially focused on the artificial intelligence and electric vehicle industries in Ohio, the pilot is creating a data system that can be expanded and applied to other industries and other states across the country.
The new tools are innovative because they build on new opportunities to produce usable information that is local, about relevant industries, and that directly tie investments in new technologies, such as AI, to labor market impacts.
Another key aspect of the NSF piloted “Industry of Ideas” is the focus on tying innovation at its source - individual data on university research activities - to the local workforce data reported by firms to their state departments of labor. The need for local data is critical because so many labor markets are local, not national in scope. Even in a global economy, many businesses and workers are locally based – as are the training providers that work to ensure that labor demand and supply are well matched. Thus the Industry of Ideas pilot provides policy makers, workers, firms, and educational institutions with access to an array of local, timely, granular, actionable resources to help them make decisions. That way, local leaders who need labor market data don’t need to rely on national unemployment figures, which are reported once a month.
Connecting science investments with jobs
The Industry of Ideas approach directly connects investment in science and the labor market, moving beyond the current approach for evaluating investment by studying scientific papers and publications 12 13 which are disconnected from workers and jobs. The data seeds were sown almost two decades ago. President Bush’s Science Advisor, John Marburger III, who, quite sensibly was unconvinced of the scientific and practical value of relying primarily on document-based, bibliometric approaches to studying science to understand its practical effects, called for a “Science of Science Policy” 14 15.
The Industry of Ideas is testing the potential to securely combine university and state data to measure the link between federal investments on local and regional economies for AI. It uses people-centric data generated by the administrative processes at universities and firms. With this data the Industry of Ideas project can capture the organization of people in science at multiple levels (e.g. individuals, teams, projects, and institutions), their multiple sources of funding (federal scientific and programmatic agencies, philanthropic foundations, industry, and state and local government), inputs into science from vendors (such as computing services, instruments, biological specimens), as well as the dynamics of their careers across time (individual career earnings and employment trajectories).
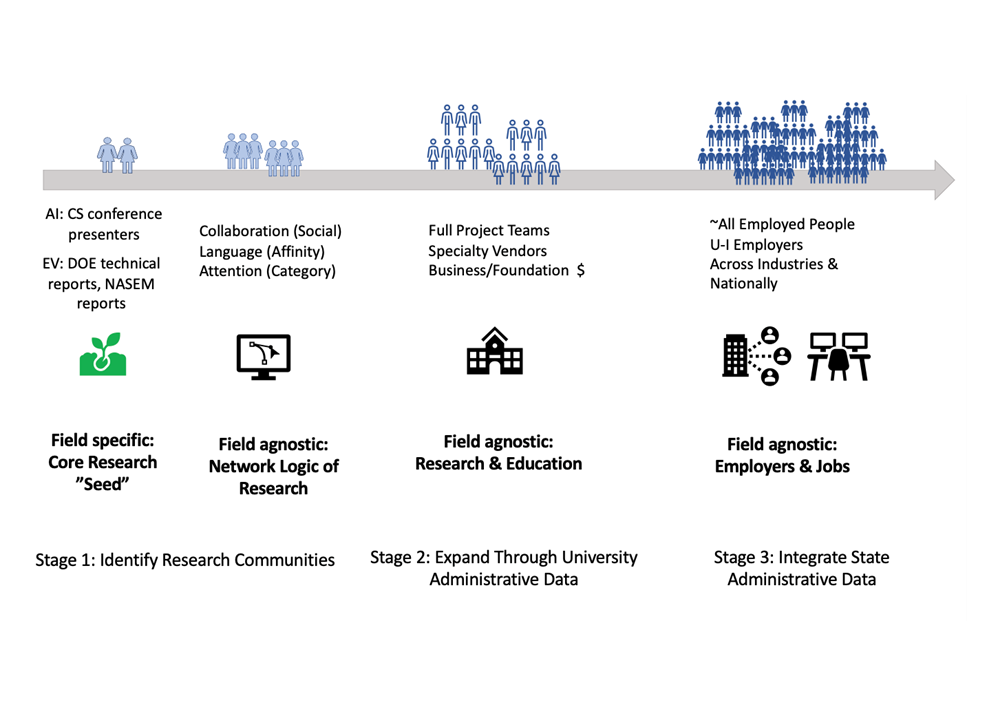
The IRIS infrastructure, developed over the past decade, provides administrative records on more than 41% of U.S. total R & D spending at universities 16. The infrastructure also provides links to survey data, as well as data from private sector suppliers 17, and can trace the flows of university funded researchers into the private sector 18 by joining up the university administrative data with state workforce data.
Tying information about AI to skills needs
How is it possible to tie changes in AI to changing needs for skills? State leaders in workforce and education agencies have identified new ways to collaborate, build staff capacity, and develop solutions, services, and products that respond to local need. An example of how to use data to get better information that more accurately connects workers with firms in the swiftly changing labor market is the New Jersey Career Navigator. It provides job seekers recommendations on new careers, available job postings, and relevant training programs based on skills similarity, labor market demand, and wage impacts observed in the underlying data. These recommendations, which are in themselvers generated by AI, show how AI technology can be used to navigate the changes in the labour market AI may cause. The New Jersey Career Navigator draws on millions of wage records, providing earnings and industry information on all workers covered by unemployment insurance in New Jersey firms; employment and wage outcomes from hundreds of thousands of graduates of occupational skills training programs in New Jersey; several years of online job postings from the National Labor Exchange Research Hub (NLx); and the resumes of 400,000 New Jersey residents.
In other words, as the Industry of Ideas pilot evolves, new ideas from states like New Jersey can be used not only to trace the flows of ideas from academia to the workplace but also to develop a new system that targets reskilling efforts once the type and location of skills needs have been identified. The new joined up data and evidence can be used to address challenges such as low labor force participation, and supplies education and training providers the data they need to align their programs with the needs of the labor market. Such a system would help government, business, educators, and workers adjust regional talent pipelines continuously in response to the changes in AI and enable workers to successfully navigate the changes that it brings.
New approaches to classifying industries: “Industries of Ideas”
An important outcome of the new NSF pilot is the potential to transform the way in which we classify firms into industries. The current industry classifications are rule based. They are designed for the economy as it was organized 40 years ago, so are not designed to describe AI. A case in point is the state of Texas – a state that anecdotally has generated a lot of high tech jobs. Current industry data for Texas is limited because firms are grouped into industries that are defined by what they produce, or how they produce it, rather than describing what new technology is being developed or utilized by those firms. As a result, the main source of labor market data in Texas provides an implausibly low picture of AI activity 19.
The Industries of Ideas approach could provide states with a new way to classify firms, based on clever new ideas of how firms can do their business, and by grouping firms by the people who created and use the technologies they will adopt 20. Examples just for Ohio include funding to use AI to improve the ways in which medicine is delivered, and advancing digital agricuture , which includes things like precision livestock farming, or precision agriculture that reduces waste and improves productivity more generally. As they interact with farmers, the clustering of university researchers and the ideas embodied in them alongside the farms that adopt those ideas represents this new type of industry cluster . Such a classification framework is a sea change from earlier industrial classifications based on what goods are physically produced - like manufacturing and agriculture 21.
The Future
Such a bottom-up classification and analysis system, based on local links between researchers and firms, could be designed locally but scaled nationally. It could address the challenges identified at the beginning of this piece. The definition of AI firms could evolve and be defined by the links between AI researchers and the firms with which they work. The lack of information about the needed AI capabilities would be resolved by the direct mapping of firm skill demand and their hiring patterns, as exemplified in New Jersey. The same New Jersey mapping could tie AI capabilities to occupational skills. And the direct impact of AI on job replacement or job augmentation could be mapped from the joined up university and workforce data.
Of course, much needs to be done. The implementation will depend on the success of the pilot, and the ability to build on existing assets. Not all states and universities have the capacity to build a similar system, but the fact that 30 universities and 15 state agencies are participating in advisory boards for the NSF Industry of Ideas pilot is grounds for hope. Indeed, a new generation of data leaders is leading the way, not only at the local and regional government level but also at universities and professional associations (Advisory Committee on Data for Evidence Building) 22.
We began this paper by noting that the urgency to create timely, local, and actionable labor market information has never been greater. We close by arguing that our capacity to fundamentally change the way in which we can use data and information to understand the demand for skills and the structure of work has also never been greater. The opportunity is ours for the taking.
- About the authors
- Julia Lane is a Professor at New York University’s Wagner Graduate School of Public Service. She was a senior advisor in the Office of the Federal CIO at the White House, supporting the implementation of the Federal Data Strategy. She recently served on two White House committees: the Advisory Committee on Data for Evidence Building and the National AI Research Resources Task Force.
-
Adam Leonard is the Chief Analytics Officer & Director of the Division of Information Innovation & Insight (I|3) for the Texas Workforce Commission (TWC). Adam envisioned and founded I|3 to help TWC leverage its most important untapped resource - its data – to help the agency and its partners better help employers, individuals, families, and communities achieve & maintain prosperity.
-
Lesley Hirsch is the Assistant Commissioner of Research and Information at the New Jersey Department of Labor and Workforce Development. Her vision for the department is to bring cutting-edge digital tools to bear to deliver labor market intelligence to the department’s internal and external customers where, when, and how they need it and to mine every data source so it can tell its full story.
- Copyright and licence
- © 2024 Royal Statistical Society
This article is licensed under a Creative Commons Attribution 4.0 (CC BY 4.0) International licence.
- How to cite
- Lane, J., Hirsch, L. and Leonard, A. 2024. “Meeting the unprecedented challenges AI poses in the labour market.” Real World Data Science, May 28, 2024. URL
Footnotes
A new approach to measuring government investment in AI-related R&D. Galindo-Rueda, F. & Cairns, S. oecd.ai (2021)↩︎
The Industry of Ideas: Measuring How Artificial Intelligence Changes Labor Markets Lane, J. AEI (2023)↩︎
NSF launches pilot to assess the impact of strategic investments on regional jobs *new.nsf.gov (2023)↩︎
AI could replace equivalent of 300 million jobs - report Vallance, C. BBC news (2023)↩︎
The Growth of Low-Skill Service Jobs and the Polarization of the US Labor Market Autor, D. H. & Dorn, D. American Economic Review 103 pp. 1553-97 (2013)↩︎
Explaining Job Polarization: Routine-Biased Technological Change and Offshoring Goos, M., Manning, A. & Salomons, A. American Economic Review 104 2509-26 (2014)↩︎
Strengthening and Democratizing the U.S. Artificial Intelligence Innovation Ecosystem Office of Science and Technology Policy (2023)↩︎
The Deep Structure of Economic Growth Romer, P. paulromer.net (2019)↩︎
Interview With Paul Romer Romer, P. & Lane, J. (2022)↩︎
Paul Romer: Ideas, nonrivalry, and endogenous growth(Jones, C. I. The Scandinavian Journal of Economics 121 859-883 (2019)↩︎
Wrapping it up in a person: Examining employment and earnings outcomes for Ph.D. recipients Zolas, N. et al. Science **350 1367-1371 (2015)↩︎
Let’s make science metrics more scientific Lane, J. Nature 464 488–489 (2010)↩︎
A Vision for Democratizing Government Data Lane, J. Issues in Science and Technology XXXIX (2022)↩︎
Let’s make science metrics more scientific Lane, J. Nature 464 488–489 (2010)↩︎
Wanted: Better Benchmarks Marburger III, J. H. Science 308 p1087(2005)↩︎
The Institute for Research on Innovation & Science (IRIS). Summary Documentation for the IRIS UMETRICS 2022 Data Release Nicholls, N., Brown, C. A., Ku, R. L. and Owen-Smith, J. D. Ann Arbor, MI: The Institute for Research on Innovation & Science (2022) doi: 10.21987/df2a-ha30↩︎
A Linked Data Mosaic for Policy-Relevant Research on Science and Innovation: Value, Transparency, Rigor, and Community Chang, W.-Y., Garner, M., Basner, J., Weinberg, B. and Owen-Smith, J. Harvard Data Science Review (2022) doi: 10.1162/99608f92.1e23fb3f↩︎
The Industry of Ideas: Measuring How Artificial Intelligence Changes Labor Markets Lane,J. American Enterprise institute (2023)↩︎
[Outside of the Box Use of Administra4ve and Wage Data in Texas] (https://digitaleconomy.stanford.edu/wp-content/uploads/2024/03/Adam-Leonard.pdf) Leonard, A. digitaleconomy.standford.edu (2024)↩︎
The Industry of Ideas: Measuring How Artificial Intelligence Changes Labor Markets Lane,J. American Enterprise institute (2023)↩︎
Converting historical industry time series data from SIC to NAICS. The Federal Committee on Statistical Methodology Yuskavage, R. Federal Committee on Statistical Methodology (2007)) – or by how services and goods are produced – like the delivery of health, financial, and investment services The Statistics Corner: The NAICS Is Coming. Will We Be Ready? Haver, M. A. Business Economics 32 63-65 (1997)↩︎
Year 2 Report Supplemental Information Advisory Committee on Data for Evidence Building (ACDEB) (2022)↩︎