“There’s some beautiful stories in clinical notes,” said Mark Sales, global strategy leader of the cloud technology company Oracle Life Sciences. He was speaking to delegates at the 2024 London Biotechnology Show about “unlocking health data and artificial intelligence within life sciences”, where opportunities abound, such as exploiting large language models (LLMs) to process some of the detailed information currently hidden in clinical notes into more structured data to inform fields like oncology. Oracle are also looking into using AI to take some of the luck out of connecting the right patients with clinical trials that might help them. The AI in Medicine and Surgery group at the University of Leeds headed by Sharib Ali has demonstrated the potential to reduce the number of times patients need to go through uncomfortable procedures like oesophageal scansfor Barrett’s syndrome , and is working on the potential to provide haptic feedback for robot mediated surgery. The London Biotechnology Showcase delegates had already heard about all these opportunities. Nonetheless Sales’s talk had opened with a note of caution: “There’s a lot more we could do, and there’s a lot more we probably shouldn’t do.”
It is an increasingly familiar caveat. “In the best scenario, AI could widely enrich humanity, equitably equipping people with the time, resources, and tools to pursue the goals that matter most to them,” suggest the Partnership on AI, a non-profit partnership of academic, civil society, industry, and media organizations. The goal of the partnership is to ensure AI brings a net positive contribution to society as a whole not just a lucky minority, which they suggest will not necessarily be the case if we rely on chance and market forces to direct progress. While people working in developing and deploying AI tackle the burgeoning size and complexity of their models, as well as the myriad requirements of testing and training data, establishing whether a model is fit for purpose, and dodging the numerous pitfalls that cause most AI projects to fail, perhaps the greatest challenge remains the range of ethical considerations including inclusiveness and fairness, robustness and reliability, transparency and accountability, privacy and security and general forethought and design. The scope of societal impact can reach far further than the immediate sphere of interaction with the model, or the interests of the companies deploying them, suggesting the need for some sort of governing forces.
However, technology is moving fast in a lot of different directions. Even with agreed sound values that all technological developments should respect, there is still space for companies to deploy AI models without supplying the necessary resources and expertise so that the roll out meets ethical and societal expectations. This expertise can range from the statistical skills required to ensure the appropriate level of representation in training datasets to the social science understanding to extrapolate potential implications for human behaviour when interacting with the technology.
Although the right checks and balances to avoid potential negative societal impacts have been slower to develop than the technologies they should be regulating, some guiding principles are emerging from organisations labouring to assess with greater clarity what the real immediate and longer term hazards are, what has worked well in other sectors, and the impact of government actions so far. There is an element of urgency in the challenge. As the Partnership on AI put it, “Our current moment serves as a profound opportunity — one that we will miss if we don’t act now.”
High stakes
When Open AI publicised their Voice Engine’s ability to clone human voices from just 15s of audio, they too flagged the potential benefit for people with poor health conditions, since those with deteriorating speech could find a means to have their speech restored. However, voice clones had already been used to make robot calls to voters imitating the voice of President Joe Biden and telling voters to stay at home.
“The question you have to ask there is what’s the societal benefit of that tool? And what are the risks,” associate director at the Ada Lovelace Institute Andrew Strait told Real World Data Science. “They thankfully decided to not fully release it,” he adds, highlighting how the timing “right before an election year with 40 democracies across the world” could have made the release particularly problematic.
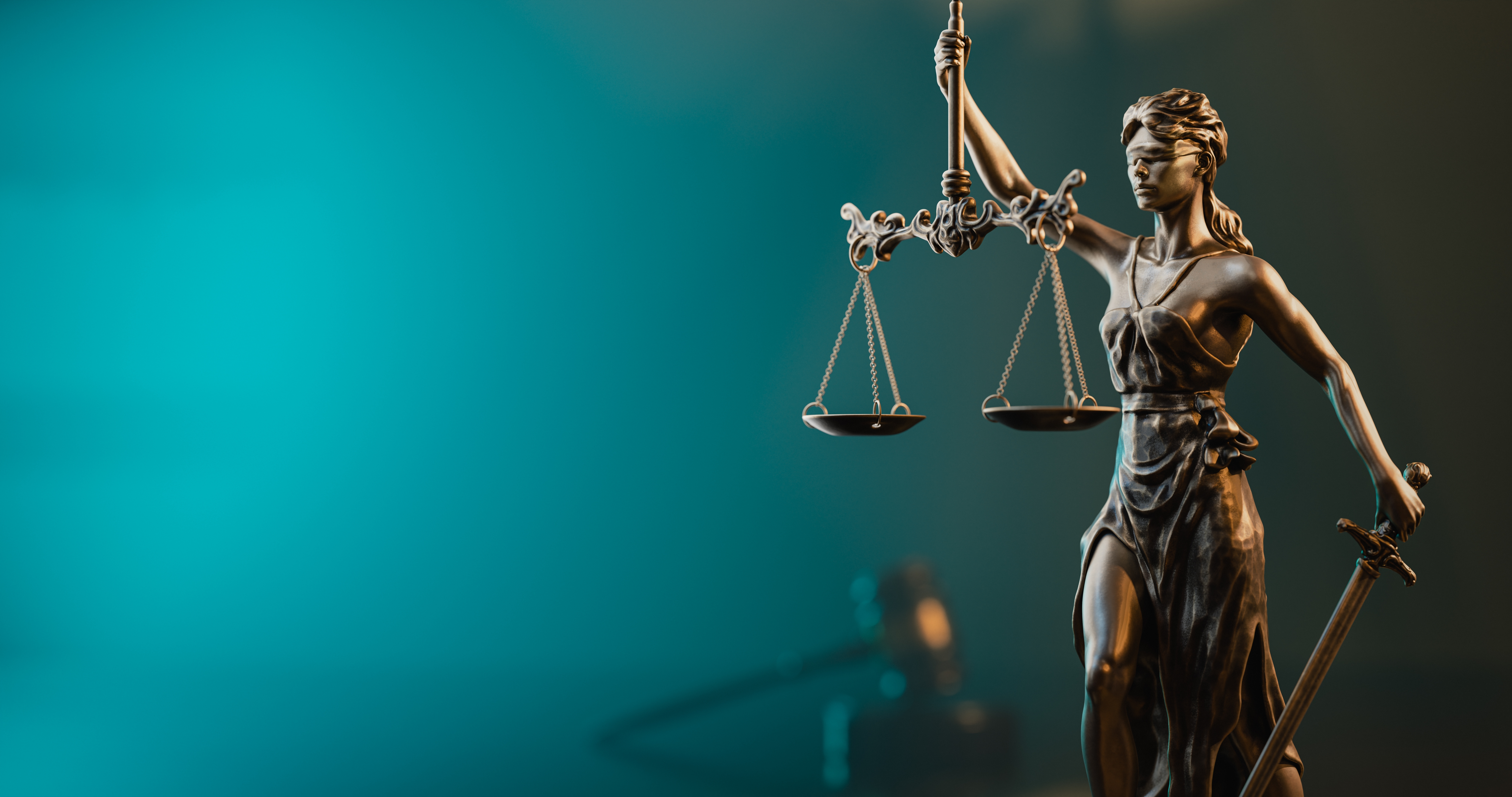
While OpenAI’s voice engine might have made voice cloning more accessible had they proceeded with a full release, voice cloning is clearly still well within reach for some already. Strait cites the experiences of hundreds of performing artists in the UK over the past few months that have been brought to the attention of the Ada Lovelace Institute. “They’re brought into a room; they’re asked to record their voice and have their face and likeness scanned; and that’s the end of their career,” says Strait. The sums paid to artists on these transactions are not large either. “They are never going to be asked to come back for audition again, because they [the companies] can generate their likeness, that voice doing anything that a producer wants without any sense of attribution, further payments, or consent to be used in that way.”
Customer service is another sector where jobs have been threatened with replacement by a generative AI chatbot, however the technology can run into problems since gen-AI is known to “hallucinate”, generating false information. Air Canada has just lost a case defending its use of a chatbot that misinformed a customer that they could apply for a bereavement fare retroactively, which is not the case according to Air Canada’s bereavement fare policy. In their defence Air Canada flagged that the chatbot had supplied a link to a webpage with the correct information but the court ruled that there was no reason to believe the webpage information over the chatbot, or for the customer to double check the information they had been supplied. While there are ways to mitigate problems with gen-AI with the right teams in place , other industries have also hit problems with the accuracy and reliability of gen-AI, which may dampen the impact AI has on the labour market. All in all the wider picture of how AI deployment may affect jobs is largely a matter of speculation. Here a US piloted scheme may soon provide framework for a more data informed approach to tackling AI’s impact on the workforce.
Strait highlights that conversations that centre around efficiency when weighing up the possible advantages of introducing AI can be ill informed. “If we’re talking about an allocation of resources in which we’re spending an increasing amount of money on automating certain parts of the NHS, or healthcare or the education system, or public sector services, how are we making the decisions that are determining if that is worth the value for money? Instead of investing in more doctors, more teachers, more social workers?” He tells Real World Data Science that these are the questions he and his colleagues at the Ada Lovelace Institute are often pushing governments to try to answer and evidence rather than to just assume the benefits will accrue. When it comes to measures of success of an AI model, Strait says “It’s often defined in terms of how many staff can be cut and still deliver some kind of service…This is not a good metric of success,” he adds. “We don’t want to just get rid of as many jobs as we can, right, we want to actually see improvements in care, improvements in service.”
Michael Katell, ethics fellow in the Turing’s Public Policy Programme and a visiting Senior lecturer at the Digital Environment Research Institute (DERI) at Queen Mary University of London suggests the problems may go deeper still when looking at the use of generative AI in creative industries. “There are definitely parallels with prior waves of disruption,” he says citing as an example the move to drum-based and eventually laser printing as opposed to manual typesetting. “A key difference, though, is that, in the creative arts, we’re talking about contributions to culture, and culture is something that, I think we often take for granted.” He highlights the often overlooked role cultural practices that enable and empower shared experiences have in holding society together. These may come in various forms from works of art to theatre, and the working and living practices among the wider community may play an important role too. While acknowledging there may be interesting and fascinating uses of AI in art to explore, Katell adds, “If we’re not attending to maintaining some aspects, or trying to manage the changes that are happening in our culture, I think we’ll see societal level effects that are much greater than the elimination of some jobs.”
The need for legislation
These stakes all highlight the need for regulatory interventions. However, most governments, bar China and the EU, have so far favoured “voluntary commitments” towards AI safety, which would seem to fall short of providing the kind of governance over the sector that can be robustly enforced. In a recent blog Strait alongside the Ada Lovelace Institute’s UK public policy lead Matt Davies and associate director (Law & Policy) Michael Birtwhistle, “evaluate the evaluations” of the UK’s AI Safety Institute for companies that have opted in for these voluntary commitments. They highlight that on the whole the companies planning to release the product hold too much control over how the evaluation can take place, ultimately empowering them to direct tests in their favour, which inhibits efforts at robust monitoring. Furthermore, there is usually no avenue for the necessary scrutiny of training data sets. Even withstanding these limitations, Davies, Strait and Birtwhistle conclude that “conducting evaluations and assessments is meaningless without the necessary enforcement powers to block the release of dangerous or high-risk models, or to remove unsafe products from the market.”
The reticence to implement firmer regulation might be attributed in some part to the perceived benefits to the state when their AI companies succeed. One often perceived benefit is that the percolating profits these companies accrue may benefit the economic buoyancy of the societies they function within. There is also cause for sovereign state competitiveness in “AI prowess” that stems from the potential for AI-based technology to underpin all aspects of society, prompting what has been described as an “AI arms race”. Here the UK may well regret allowing Google to acquire Deep Mind, whose output is responsible for bolstering the “UK’s share” of citations in the top 100 recent AI papers from 1.9% to 7.2%. However, a lack of robust regulation may prove as much a disservice to the companies releasing AI products as it is to society as a whole.
“The medicine sector here [in the UK] is thriving, not in spite of regulation, but because of regulation,” says Strait. “People trust that the products you develop here are safe.” Katell, highlights the impact of pollution legislation on the automotive industry. “It jumped forward invention and discovery in automotive technology,” he tells Real World Data Science. “It seems prosaic in hindsight, but it wasn’t, it was a major innovation that was promoted by regulators, promoted by legislators.” The UK government’s chief scientific advisor Angela McLean seems to agree. “Good regulation is good for innovation,” she replied when asked about balancing regulation with favourable conditions for a flourishing AI sector at an Association of British Science Writers’ event in May. “We’re not there yet,” she added. The challenge is pinning down what good regulation looks like.
Regulatory ecosystems
As has been emphasised throughout the series, making a success of an AI project requires a unique skillset that combines expertise in AI with the domain expertise for the sector the project is contributing to, and there is often a dearth of people that straddle both camps. The same hunt for “unicorns” with useful expertise in the tech sector and policymakers can also be an obstacle for developing “good regulation”. One solution is to bring people from the different disciplines together to develop legislation collaboratively, as was arguably the case with the roll out of General Data Protection Regulations (GDPR) in 2018. “Policymakers and academics, they worked very closely together in the crafting of that law,” says Katell. “It was one of those rare moments in which we saw the boundaries really dissolve between policy and academia in a way that delivered something that I think we can agree was largely a positive outcome.”
When it comes to AI, an obstacle to that kind of collaboration has been the lack of a common language. In “Defining AI in policy and practice” in 20201, Katell alongside Peaks Krafft at the University of Oxford and co-authors found that AI researchers favoured definitions of AI that “emphasise technical functionality”, whereas policy-makers tended towards definitions that “compare systems to human thinking and behavior”, which AI systems remain far from achieving. Strait also highlights a recurring theme among those without experience of actually making AI systems in overselling AI capabilities in suggestions that it will “help solve climate change” or “cure cancer”. “How are you measuring that?” he asks. “How are we making a clear sense of the efficacy, the proof behind those kinds of statements? Where are the case studies that actually work, and how are we determining that’s working?”
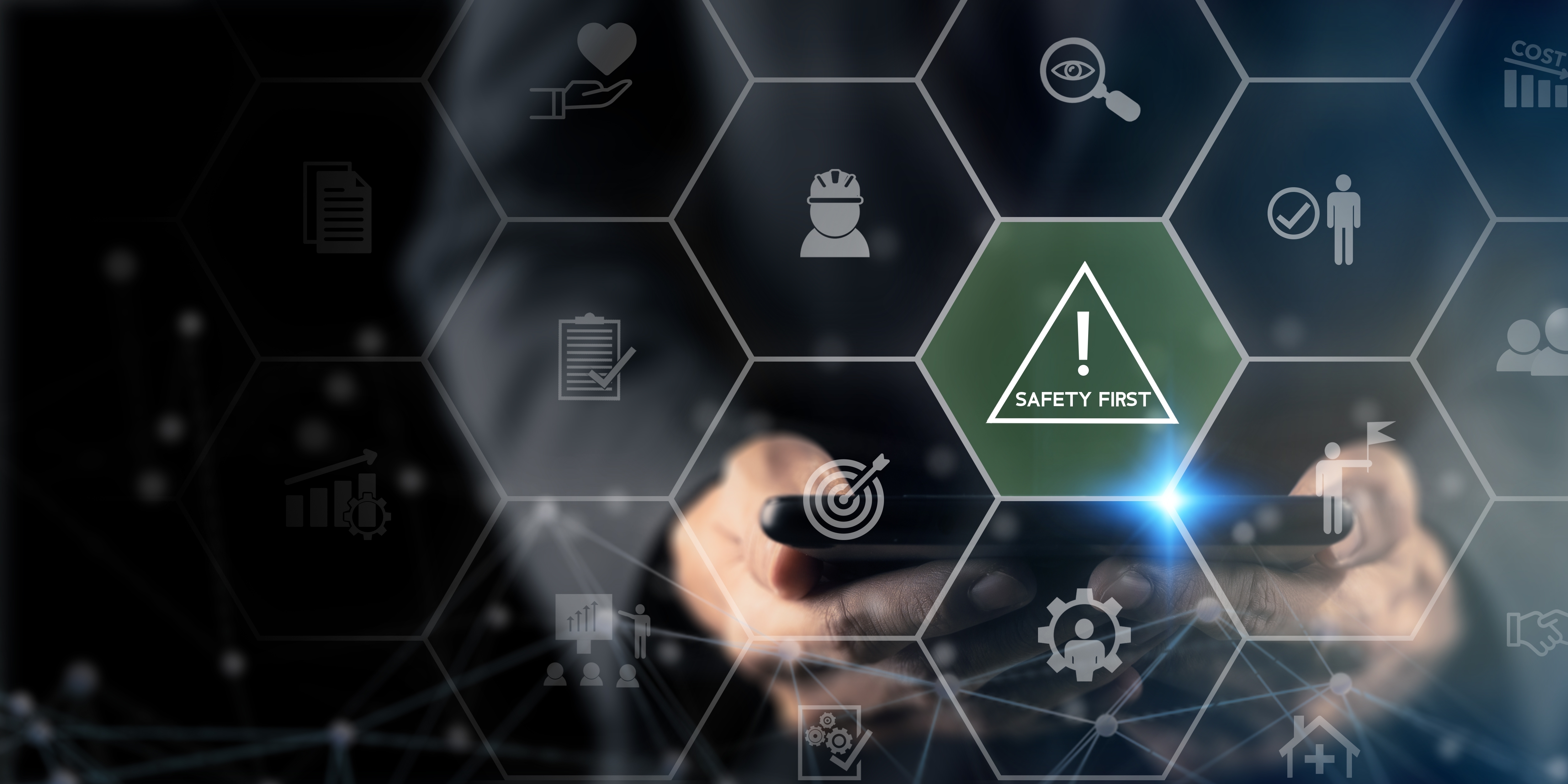
As Krafft et al. point out in their 2020 paper, such exaggerated perceptions of AI capabilities can also hamper regulation. “As a result of this gap,” they write, “ethical and regulatory efforts may overemphasise concern about future technologies at the expense of pressing issues with existing deployed technologies.” Here a better understanding of what AI is can be helpful to focus attention on the problems that exist now – not just the potential workforce impact, but the carbon cost of training large language models, activities like nonconsensual gen-AI porn aggravating online gender inequality, and a widening digital divide disadvantaging pupils, workers and citizens who cannot afford all the latest AI tools, among others.
Fortunately, there has already been progress to breach the language divide between policy makers and the tech sector. “The current definitions [championed in policy circles] say things like technologies that can perform tasks that require intelligence when humans do them,” says Katell, which he describes as a far more sober and realistic definition than likening technologies to the way humans think and work. “This is really important,” he adds. “Because some of the problems that we see with AI now are symptomatic of the fact that they’re not humans and that they don’t have the same experience of the world.” As an example he describes someone driving a car with child in the car seat, calling on all their training and experience of road use to navigate roads and other traffic, while juggling their attention between driving and the child. “Things that AI is too brittle, to accomplish,” he adds, highlighting how a simple model may identify school buses in images quite impressively until it is presented with an image of a bus upside down. “The flexibility and adaptability, the softness of human reason, is actually its strength, its power.”
Getting everybody on the same page can also help provide a more multimodal approach to governance. Empowering independent assessors of AI product safety prior to release is one thing but as Strait points out, “It could be more like the environmental sector, where we have a whole ecosystem of environmental impact assessments, organisations and consultancies that do this kind of work for different organisations and companies.” Internal teams within companies can play an important role too so long as they work sufficiently independently from the companies themselves. When set up with the right balance of expertise they can be better placed to understand and hence assess the technology and practical elements of its implementation. Although such teams can be expensive, getting the technical evaluation and consideration of ethical issues right can pose a competitive advantage for the companies themselves as well as providing a more thorough safeguard for society at large. Nonetheless there are also obvious advantages in having external regulatory bodies, which do not need to take into account the company’s profit margins or shareholders’ needs. An ideal set up might incorporate both approaches. In fact in their appraisal of the current UK AI Safety Institute arrangement, Davies, Strait and Birtwistle first highlight the need to integrate the AI Safety Institute “into a regulatory structure with complementary parts that can provide appropriate, context-specific assurance that AI systems are safe and effective for their intended use.”
Prosperity for all
With all the precedents in other sectors from environmental impact checks to pharmacology, an organised framework or ecosystem for robust, independent and meaningful evaluation of AI product safety seems an inevitable imperative, albeit potentially expensive. (Davies, Strait and Birtwistle cite £100 million a year as a typical cost for safety driven regulatory systems in the UK2, and the expertise demands of AI could further increase costs.) However, such regulatory reform will likely slow down the pace of technological development and the route to market. While the breathing space to adjust to the societal changes they bring with them may be welcomed by some, the delay can be quite unpopular in a tech sector where the ethos is famed for embracing a “move fast, break things” mentality. As Katell points out that ideal is based on the notion that the things being broken were unimportant – when it’s vulnerable people and societies that is “unacceptable breakage”.
Strait also highlights the cultural mismatch between the companies developing AI products – where the research to market pipeline is extremely fast – and the sectors those tools are intended to serve, such as social care, education and health. Although Open AI eventually decided against full release of the Voice Engine, when it comes to the ethos of some AI technology companies , “The default is to put things out there and to not think through the ethical and societal implications,” says Strait who has direct experience of working for a company producing AI tools in the past. “I think it’s so critical for data scientists and ethicists to explore, and do that translation and interrogation of what are the ethics of the sector that we’re working in?”
Katell voices concern shared by many that at present AI is under the control of a very small handful of very large, powerful technology companies, and as a result the AI releases making the most impact are targeting the agendas of the companies releasing them and their current and anticipated customer base, as opposed to the needs of society. The potential for such large tech agents to become too big to fail poses additional regulatory challenges. While many may lament the tension between a demand for open source data sets for testing AI models versus the need to respect data privacy, security and confidentiality, there have already been widely mooted instances where certain companies may not have met expectations for respecting copyright and terms of service. In fact the tech giants are not the only people developing AI models and the open source community have been known to pose valuable competition that may temper the tendency for AI to concentrate a lot of power into the hands of a small few3. However, open source developers can also pose a certain amount of regulatory complexity.
There is also an argument that these efforts should broaden their scope beyond baseline AI safety and aim to focus efforts in AI development towards tools that actively promote greater wellbeing and prosperity to the many. “We need to bring in other values like fairness, justice, and simple things like explainability, gender equity, racial equity,” says Katell, highlighting some of the other qualities that demand attention among others. Taking explainability as an example, there is increasing awareness of the need to understand how certain outputs are reached in order for people to feel comfortable with the technology, and the outputs requiring explanations differ from person to person. Although it can be hard to explain AI outputs, progress is being made in this direction. As Katell says, “We’re not helpless in managing these types of disruptions. It’s a matter of societies coming together and deciding that they can be managed.”
- About the authors
- Anna Demming is a freelance science writer and editor based in Bristol, UK. She has a PhD from King’s College London in physics, specifically nanophotonics and how light interacts with the very small, and has been an editor for Nature Publishing Group (now Springer Nature), IOP Publishing and New Scientist. Other publications she contributes to include The Observer, New Scientist, Scientific American, Physics World and Chemistry World.
- Copyright and licence
- © 2024 Royal Statistical Society
This article is licensed under a Creative Commons Attribution 4.0 (CC BY 4.0) International licence.
- How to cite
- Demming, Anna. 2024. “Ensuring new AI technologies help everyone thrive .” Real World Data Science, June 11, 2024. URL
References
Krafft, P. M., Young, M., Katell, M., Huang, K. & Bugingo, G. Defining AI in Policy versus Practice AIES ’20: Proceedings of the AAAI/ACM Conference on AI, Ethics, and Society 72-78 (2020)↩︎
Smakman, J, Davies, M. & Birtwhistle, M. Mission critical Ada Lovelace Policy Briefing (2023)↩︎
Google “We Have No Moat, And Neither Does OpenAI semianalysis.com (2023) (semianalysis.com)↩︎